Interdisciplinary team approach to improve stroke care by applying AI to imaging.
Stroke is one of the leading causes of death worldwide. Morbidity is high, with over half of stroke victims left chronically disabled. Dr. Jennifer Soun, an Assistant Professor in Radiological Sciences at UCI, is working closely with the Center for Artificial Intelligence in Diagnostic Medicine (CAIDM) and the stroke interdisciplinary team consisting of neurologists, neurointerventionalists, and radiologists to help validate and deploy AI-based tools for stroke triage.
Recent clinical trials in the extended time window have shown promising outcomes for thrombectomy, with the approximate number needed to treat for revascularization to show clinical benefit as less than 3 . Thus, establishing criteria to allow for more patients to benefit from treatment is of strong clinical interest. In recent years, there has been a shift from the singular paradigm of “time is brain” to a model incorporating various physiologic factors such as collateral status, which likely is a major determinant of revascularization rates, tissue fate, and extended window eligibility. However, current grading systems rely on heterogeneous non- standardized methods and subjective visual inspection which limit uniform application of collateral assessment in stroke treatment decision- making. Our group has recently developed a comprehensive deep learning-based cerebrovascular tracing tool for detection of proximal vessel occlusion. We plan adapt the algorithm to quantify collateral assessment on CT angiography (CTA) and identify phenotypic collateral patterns predictive of imaging and clinical outcomes (Figure 1).
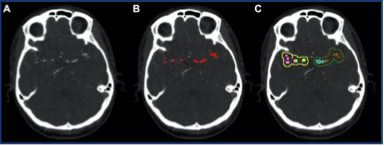
Figure 1: A 3D deep learning AI tool for vascular segmentation on CTA.
Our group has also developed AI tools to detect intracerebral hemorrhage (ICH). One particular area of interest is in ICH expansion which has been shown to be an independent predictor of mortality and functional outcome, with each milliliter of expansion increasing the chance of functional dependence by up to 7%. However, detection of ICH expansion can be inaccurate and subjective based on current methods, and thus may misguide treatment decisions. One deep learning AI-based tool that UCI radiologists created is able to detect acute intracranial hemorrhage expansion with >99% accuracy and outperforms a traditional ABC/2 estimative approach (Figure 2). In addition, the AI- based tool is much faster at estimating ICH volume compared to a human reader. This work was presented at the 2020 International Stroke Conference, and our next step is application in the ER and inpatient setting in a prospective study.

Figure 2: Quantification of a spontaneous ICH. Segmented volume was 12 cm3 by CNN compared to 22 cm3 by ABC/2.
These AI tools leverage the accuracy, speed, and quantitative nature of deep learning approaches to noninvasively and objectively characterize various components of the stroke triage pathway. In the future, UCI radiologists hope to deploy these tools in the clinical work setting to aid in treatment selection and improvement of clinical outcomes.
Jennifer Soun, MD